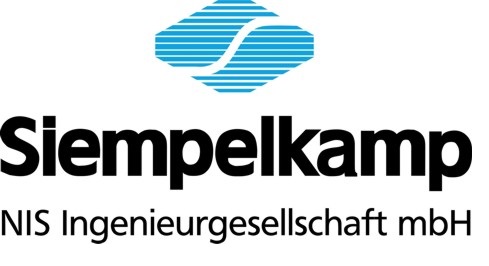
Initial situation
Siempelkamp NIS Ingenieurgesellschaft mbH provides monitoring solutions for large steam and gas turbine generator sets in the energy industry with outputs ranging from 50 MW to 1000 MW. For these plants, a monitoring concept based on high-resolution vibration measurement and analysis has been developed over many years. To detect deviations from the normal operation mode and prevent damage, tolerance bands are currently determined using computational methods and optimized by experts. Exceedances of these tolerance bands are detected by the software and evaluated manually.
Problem definition
In the course of the renewable energy transition, large turbines will lose importance and energy generation will shift to smaller, decentralized industrial turbines. This will necessitate a reduction in manual data evaluation. Therefore, the QuickCheck project investigated to what extent an AI/ML solution can be developed to automatically monitor the turbine behavior.
Solution
From a ML point of view, the problem can be seen as a form of anomaly detection. In the course of the QuickCheck, this approach was investigated using two data sets, one representing the normal operation mode and one representing the fault operation mode. For the analysis, a machine learning model was trained on the data in normal operation and validated against a data set containing a disturbance. Using the trained model, it was possible to correctly identify the malfunction occurring in the process and draw a conclusion about the cause of the fault using the relevant sensors.
QuickCheck results
The results of the QuickCheck have shown that using an ML driven assistance system, monitoring of the turbine under consideration can be realized: Faults are reliably detected and causes of faults can be identified with the help of experts. The QuickCheck has led to encouraging results on the part of the use of an AI system for turbine monitoring. The QuickCheck also considered possible options for a system architecture to implement the AI methodology in spatially remote turbines and evaluated it in terms of scalability and maintainability of the AI.